How well do AI models like GPT-4 understand XBRL Data?
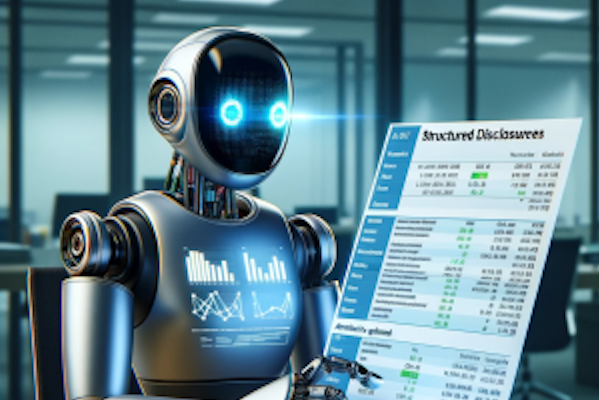
A couple of weeks ago we commented unfavourably on the use of PDF versions of SEC filings as inputs to Large Language Models (LLMs). We are much more interested in how these tools can be leveraged to examine structured data.
Examples include the Inline XBRL formatted structured filings provided to the SEC by every listed company in the United States.
So we thought we should do some experimentation of our own. In a new blog, our Guidance Manager, Revathy Ramanan dives into what’s possible with some very simple approaches to LLM-based analysis that relies on structured XBRL financial statements rather than unstructured PDFs.
This blog explains our process, and sets out some initial, tentative findings. We used Chat GPT 4 for this work (even XBRL International can afford it). We are, to be honest, much more interested in what you can do with these tools. It feels like a whole new chapter is opening up, but a wide range of experimentation, using bespoke LLMs, is going to be needed.
In a nutshell, the more we do in this space, the more we are convinced that trusted, structured data disclosures in XBRL — that management is accountable for — produces a single source of truth and an analytical goldmine for artificial intelligence. Data is everywhere. Trusted data is priceless. Of course, the fact that it can be easily consumed by AI is very positive, but we are still at the earliest point in this journey. Will you take the next step?
Food for thought! You can read the full results and follow the experiment (and perhaps be inspired to explore using LLM’s on XBRL data yourself) on our blog.