Why structured data leaves PDFs far behind in AI analysis – and the power of XBRL taxonomies
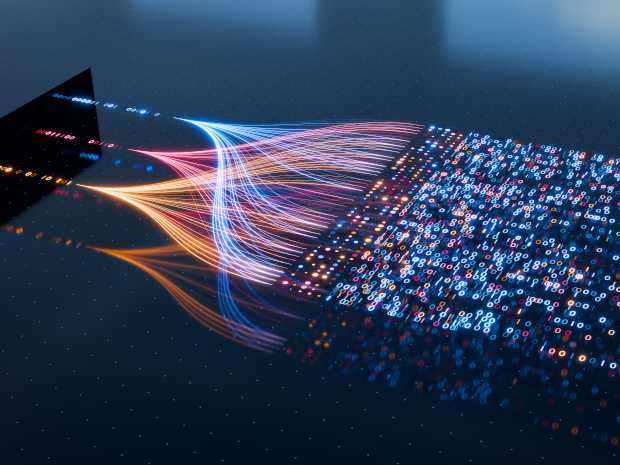
Large language models (LLMs) like ChatGPT are transforming the way people work across the globe – and we’re all keen to know what they can do with XBRL reports. XBRL International Guidance Manager Revathy Ramanan continues her occasional blog series this week, exploring why structured data outperforms PDFs in LLM analysis, particularly in combination with taxonomy metadata.
In her experiments, Revathy shows how information on taxonomy relationships can help ChatGPT identify unusual practices or potential errors in disclosures. She also demonstrates how we can leverage taxonomy references to locate and extract data with efficiency and precision – a critical advantage for anyone interested working in analytics. On the other hand, she illustrates the shortcomings of PDFs for LLM analysis, particularly the propensity of ChatGPT to incorrectly capture and incorporate irrelevant information from nearby report sections.
As we move into the new year, why not try experimenting with AI yourself? We have an exciting and unprecedented opportunity to leverage the power of LLMs in combination with structured XBRL data and accompanying taxonomies – maximising the reach and power of analytics to provide insights on performance, identify anomalies, and highlight emerging risks. At the same time, AI tools look set to enhance disclosure quality and consistency, offering the vision of a virtuous cycle of high-value data and powerful analysis. No doubt we’ll be bringing you much more news and many additional ideas on all of this in 2025!
Read the article here, or watch her original Data Amplified 2024 presentation here.